- All Templates
- Category: data science
- Category: business
- Category: analytics
- Data Science Maturity Matrix
Data Science Maturity Matrix
The Data Science Maturity Matrix is a strategic tool used to assess and visualize the maturity level of an organization's data science capabilities. It helps businesses identify their current stage, ranging from initial data collection to advanced predictive analytics, and provides a roadmap for growth and improvement.
At a very high level, the Data Science Maturity Matrix is used in the context of business, data science, analytics.
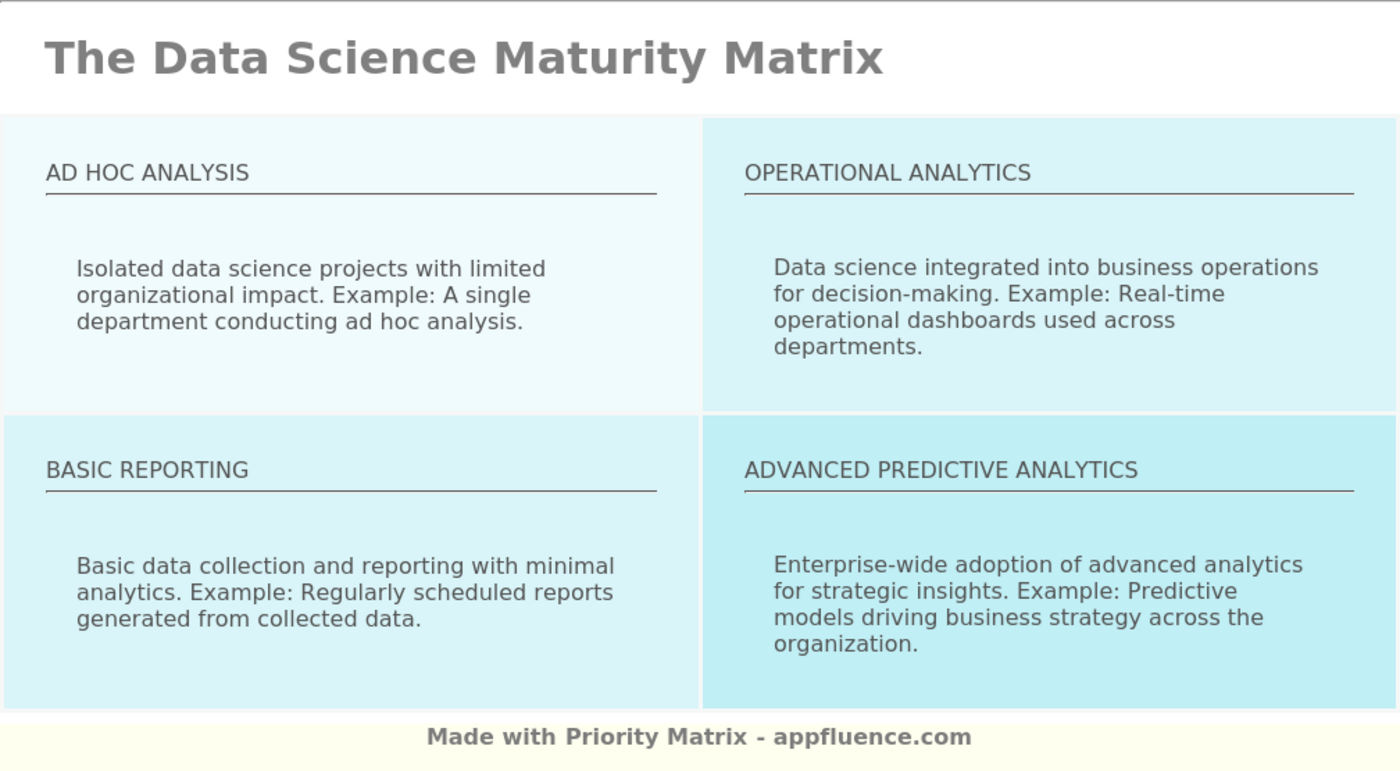
Want to try this template?
Other Templates
- Product-Market Matrix
- 4 Ps Marketing Mix Matrix
- AI Capability-Value Proposition Alignment Matrix
- AI Innovation-Value Alignment Matrix
- AI Maturity Matrix
- AI-Value Proposition Alignment Matrix
- AI-Value Proposition Matrix
- AIDA Marketing Matrix
- 4 Ps Marketing Mix Matrix
- Action Priority Matrix
- ADKAR Change Management Model
- Adoption Curve Matrix
- Advertising Effectiveness Matrix
- Advertising Message-Target Audience Matrix
- Affiliate Marketing Matrix
- Agile Capability Assessment-Value Matrix
- Agile Capability-Value Alignment Matrix
- Agile Development-Cost Matrix
- Agile Development-Performance Matrix
- Agile Maturity Matrix
- Agile Maturity-Performance Alignment Matrix
- Agile Methodology Capability-Performance Alignment Matrix
- Agile Methodology Maturity Matrix
- Agile Methodology-Performance Alignment Matrix
- Agile Planning Matrix
- Agile Product Roadmap Matrix
- Agile Release Train Matrix
- Agile Scaling Matrix
- Agile Transformation Matrix
- Agile Value Proposition Matrix
- Agile Value Stream Mapping Capability Maturity Matrix
- Agile Value Stream Mapping Matrix
- Agile Workforce Capability Maturity Matrix
- AI Capability-Value Proposition Alignment Matrix
- AI Innovation-Value Alignment Matrix
- AI Maturity Matrix
- AI-Value Proposition Alignment Matrix
- AI-Value Proposition Matrix
- AIDA Marketing Matrix
- Ansoff Matrix
- Artificial Intelligence-Value Chain Alignment Matrix
- Asset Allocation Matrix
- Asset-Liability Management Matrix
- Audience Segmentation Matrix
- Awareness-Interest-Desire-Action Matrix
- Balanced Business Scorecard Matrix
- Balanced Scorecard
- Balanced Scorecard Strategy Map Matrix
- BCG Matrix
- Behavioral Mapping Matrix
- Behavioral Segmentation Matrix
- Benefit-Cost Analysis Matrix
- Benefits Analysis Matrix
- Benefits Management Matrix
- Benefits Realization Matrix
- Blockchain Adoption Matrix
- Bottom of the Funnel Matrix
- Brand Affinity Matrix
- Brand Association Matrix
- Brand Awareness Matrix
- Brand Differentiation Matrix
- Brand Equity-Performance Alignment Matrix
- Brand Extension Matrix
- Brand Loyalty Matrix
- Brand Messaging-Consumer Perception Matrix
- Brand Perception-Performance Matrix
- Brand Perception-Value Matrix
- Brand Personality-Consumer Perception Matrix
- Brand Positioning Matrix
- Brand Purpose-Consumer Perception Matrix
- Brand Reputation-Performance Alignment Matrix
- Brand Reputation-Value Matrix
- Brand Resonance Matrix
- Brand Salience Matrix
- Brand Strategy-Value Proposition Alignment Matrix
- Brand Switching Matrix
- Brand-Consumer Relationship Matrix
- Business Analysis Matrix
- Business Architecture Matrix
- Business Capability Assessment-Value Matrix
- Business Capability Assessment-Value Stream Alignment Matrix
- Business Capability Assessment-Value Stream-Value Chain Alignment Matrix
- Business Capability Matrix
- Business Capability Maturity Assessment-Performance Matrix
- Business Capability Maturity Matrix
- Business Capability-Value Stream Alignment Matrix
- Business Continuity Management Matrix
- Business Decision-Making Matrix
- Business Ecosystem Matrix
- Business Ecosystem Value Matrix
- Business Impact Analysis Matrix
- Business Impact Matrix
- Business Impact-Probability Matrix
- Business Intelligence Matrix
- Business Intelligence Maturity Matrix
- Business Model Canvas
- Business Model Innovation Matrix
- Business Model Matrix
- Business Model-Value Matrix
- Business Opportunity Matrix
- Business Opportunity-Performance Alignment Matrix
- Business Performance-Value Matrix
- Business Process Improvement Matrix
- Business Process Improvement-Value Matrix
- Business Process Management Capability Assessment Matrix
- Business Process Management Matrix
- Business Process Mapping Matrix
- Business Process Matrix
- Business Process Maturity Assessment Matrix
- Business Process Optimization-Value Alignment Matrix
- Business Process Simulation-Value Alignment Matrix
- Buyer Decision-Making Process Matrix
- Buying Center Matrix
- Capability Assessment and Planning Matrix
- Capability Assessment Matrix
- Capability Development Matrix
- Capability Gap Matrix
- Capability Heat Map
- Capability Maturity Assessment Matrix
- Capability Maturity Level Matrix
- Capability Maturity Level-Value Matrix
- Capability Maturity Model Integration (CMMI)
- Capability Maturity Model Integration (CMMI)
- Capability Maturity Model Integration Matrix
- Capability Planning Matrix
- Capability Prioritization Matrix
- Capability Readiness Matrix
- Capability-Based Assessment Matrix
- Capability-Based Budgeting Matrix
- Capability-Based Investment Matrix
- Capability-Based Planning Matrix
- Capability-Based Risk Assessment Matrix
- Capability-Performance Alignment Matrix
- Capability-Performance-Value Stream Alignment Matrix
- Capability-Technology Matrix
- Capability-Technology Maturity Matrix
- Capability-Value Chain Matrix
- Capability-Value Matrix
- Capability-Value Proposition Matrix
- Capability-Value Proposition-Value Chain Alignment Matrix
- Capability-Value Proposition-Value Stream Alignment Matrix
- Capability-Value Proposition-Value Stream-Value Chain Alignment Matrix
- Capability-Value Proposition-Value Stream-Value Chain-Performance Alignment Matrix
- Capability-Value Stream Matrix
- Capability-Value-Complexity Matrix
- Capability-Value-Maturity Matrix
- Capability-Value-Probability Matrix
- Capability-Value-Readiness Matrix
- Change Management Impact Matrix
- Change Management Maturity Matrix
- Channel Effectiveness Matrix
- Channel Management Matrix
- Channel Mix Matrix
- Channel Partner Capability Assessment-Performance Alignment Matrix
- Channel Partner Capability Assessment-Value Matrix
- Channel Partner Capability Maturity Matrix
- Channel Partner Performance-Value Alignment Matrix
- Channel Partner Performance-Value Stream Alignment Matrix
- Channel Strategy Matrix
- Churn Matrix
- Clickstream Analysis Matrix
- Cloud Computing Adoption Matrix
- Cloud Migration Risk-Value Matrix
- Cloud Readiness Assessment Matrix
- Cloud Readiness-Value Alignment Matrix
- Cloud Security Risk-Value Alignment Matrix
- Cloud Security-Value Alignment Matrix
- Cloud Service Performance-Value Alignment Matrix
- Collaborative Work Environment Matrix
- Competency Assessment Matrix
- Competency Assessment-Value Matrix
- Competency Capability Maturity Matrix
- Competency Capability-Value Matrix
- Competency Development-Value Alignment Matrix
- Competency Mapping-Value Alignment Matrix
- Competency Matrix
- Competency-Based Interview Matrix
- Competency-Based Performance Matrix
- Competency-Value Matrix
- Competitive Advantage Matrix
- Competitive Advantage-Value Matrix
- Competitive Advantage-Value Proposition Alignment Matrix
- Competitive Analysis Matrix
- Competitive Assessment Matrix
- Competitive Environment-Size Matrix
- Competitive Position-Value Proposition Alignment Matrix
- Competitive Strategy Matrix
- Compliance Risk-Value Matrix
- Concept Testing Matrix
- Configuration Management Matrix
- Constraint Analysis Matrix
- Consumer Behavior-Value Alignment Matrix
- Consumer Behavior-Value Stream Alignment Matrix
- Consumer Decision-Making Matrix
- Consumer Insight Matrix
- Consumer Insights-Performance Matrix
- Consumer Insights-Value Matrix
- Consumer Insights-Value Proposition Alignment Matrix
- Content Creation Matrix
- Content Marketing Matrix
- Continuous Improvement Matrix
- Conversion Rate Optimization Matrix
- Core Competency Matrix
- Cost-Volume-Profit Analysis Matrix
- Critical Success Factors Matrix
- Customer Acquisition-Cost Matrix
- Customer Acquisition-Retention Matrix
- Customer Acquisition-Retention-Cost Matrix
- Customer Analysis Matrix
- Customer Analysis-Insight Matrix
- Customer Analysis-Value Matrix
- Customer Analytics-Performance Alignment Matrix
- Customer Analytics-Value Matrix
- Customer Analytics-Value Stream Alignment Matrix
- Customer Behavior-Performance Matrix
- Customer Behavior-Value Chain Alignment Matrix
- Customer Behavior-Value Matrix
- Customer Churn Rate Matrix
- Customer Churn-Value Matrix
- Customer Contact Matrix
- Customer Effort-Value Matrix
- Customer Emotion Matrix
- Customer Engagement Matrix
- Customer Experience Journey Matrix
- Customer Experience Maturity Matrix
- Customer Experience-Mapping Matrix
- Customer Experience-Value Chain Alignment Matrix
- Customer Experience-Value Matrix
- Customer Experience-Value Proposition Alignment Matrix
- Customer Experience-Value Proposition-Value Chain Alignment Matrix
- Customer Experience-Value Stream Alignment Matrix
- Customer Experience-Value Stream-Value Chain Alignment Matrix
- Customer Feedback-Insight Matrix
- Customer Feedback-Performance Matrix
- Customer Feedback-Value Matrix
- Customer Insight Matrix
- Customer Insight-Opportunity Matrix
- Customer Journey Mapping Matrix
- Customer Journey-Experience Matrix
- Customer Journey-Mapping Matrix
- Customer Journey-Maturity Matrix
- Customer Lifetime Value-Cost Matrix
- Customer Lifetime Value-Experience Matrix
- Customer Lifetime Value-Value Matrix
- Customer Lifetime Value-Value Matrix
- Customer Loyalty Matrix
- Customer Loyalty-Experience Matrix
- Customer Loyalty-Value Matrix
- Customer Needs Analysis Matrix
- Customer Needs Matrix
- Customer Needs-Opportunities Matrix
- Customer Persona Matrix
- Customer Persona-Value Matrix
- Customer Personas Matrix
- Customer Relationship Management Matrix
- Customer Retention-Performance Matrix
- Customer Satisfaction and Loyalty Matrix
- Customer Satisfaction Matrix
- Customer Satisfaction-Insight Matrix
- Customer Satisfaction-Performance Alignment Matrix
- Customer Satisfaction-Value Alignment Matrix
- Customer Satisfaction-Value Proposition Alignment Matrix
- Customer Segmentation and Targeting Matrix
- Customer Segmentation and Targeting Matrix
- Customer Segmentation-Analysis Matrix
- Customer Segmentation-Opportunity Matrix
- Customer Segmentation-Value Alignment Matrix
- Customer Segmentation-Value Analysis Matrix
- Customer Segmentation-Value Chain Alignment Matrix
- Customer Segmentation-Value Matrix
- Customer Service-Value Matrix
- Customer Value Matrix
- Customer Value Proposition Matrix
- Customer Value-Insight Matrix
- Customer Value-Opportunity Matrix
- Customer Value-Performance Alignment Matrix
- Customer Value-Readiness Matrix
- Customer Value-Value Proposition Alignment Matrix
- Customer Value-Value Stream-Value Chain Alignment Matrix
- Cybersecurity Risk Matrix
- Data Analysis-Performance Matrix
- Data Analytics Capability Assessment Matrix
- Data Analytics Matrix
- Data Governance Maturity Matrix
- Data Management Capability Matrix
- Data Management Capability-Value Alignment Matrix
- Data Management Capability-Value Proposition Alignment Matrix
- Data Management Maturity Assessment Matrix
- Data Quality-Cost Matrix
- Data Quality-Performance Matrix
- Data Science Maturity Matrix
- Data Value Matrix
- Decision Analysis Matrix
- Decision Matrix
- Delphi Method Matrix
- Design Thinking Matrix
- Digital Adoption Matrix
- Digital Capability Assessment Matrix
- Digital Capability Assessment-Value Alignment Matrix
- Digital Capability Matrix
- Digital Capability Maturity Assessment Matrix
- Digital Capability Maturity Assessment-Performance Alignment Matrix
- Digital Capability Maturity Assessment-Value Proposition Alignment Matrix
- Digital Capability-Performance Alignment Matrix
- Digital Channel Effectiveness Matrix
- Digital Engagement Matrix
- Digital Experience Matrix
- Digital Innovation Matrix
- Digital Marketing Effectiveness Matrix
- Digital Marketing Matrix
- Digital Marketing Maturity Matrix
- Digital Maturity Assessment-Performance Matrix
- Digital Maturity Assessment-Value Alignment Matrix
- Digital Maturity Matrix
- Digital Maturity-Competitiveness Matrix
- Digital Maturity-Performance Matrix
- Digital Maturity-Value Alignment Matrix
- Digital Strategy Matrix
- Digital Strategy-Value Matrix
- Digital Transformation Capability Assessment Matrix
- Digital Transformation Capability Maturity Matrix
- Digital Transformation Capability-Performance Alignment Matrix
- Digital Transformation Capability-Value Alignment Matrix
- Digital Transformation Impact Matrix
- Digital Transformation Impact-Readiness Matrix
- Digital Transformation Impact-Value Matrix
- Digital Transformation Matrix
- Digital Transformation Maturity Matrix
- Digital Transformation Performance-Value Alignment Matrix
- Digital Transformation Performance-Value Stream Alignment Matrix
- Digital Value Chain Matrix
- Digitalization Maturity Matrix
- Digitalization Performance-Value Matrix
- Digitalization Readiness Matrix
- Direct Marketing Matrix
- Disaster Recovery Matrix
- Distribution Channel Matrix
- Diversity and Inclusion Matrix
- Diversity and Inclusion Matrix
- Due Diligence Matrix
- Earned Value Management Matrix
- Economic Value Added Matrix
- Effort Impact Matrix
- Emotional Appeal Matrix
- Emotional Intelligence Matrix
- Emotional Labor Matrix
- Employee Capability Assessment Matrix
- Employee Capability Assessment-Development Matrix
- Employee Capability Development-Performance Alignment Matrix
- Employee Capability Development-Value Alignment Matrix
- Employee Capability Development-Value Proposition-Value Chain Alignment Matrix
- Employee Capability-Value Chain Alignment Matrix
- Employee Capability-Value Matrix
- Employee Capability-Value Proposition Alignment Matrix
- Employee Development Matrix
- Employee Development-Value Matrix
- Employee Engagement Matrix
- Employee Performance Matrix
- Employee Performance-Development Matrix
- Employee Performance-Value Alignment Matrix
- Employee Performance-Value Chain Alignment Matrix
- Employee Performance-Value Proposition Alignment Matrix
- Employee Performance-Value Proposition-Value Stream Alignment Matrix
- Employee Performance-Value Stream Alignment Matrix
- Employee Performance-Value Stream-Value Proposition Alignment Matrix
- Employee Performance-Value-Engagement Matrix
- Employee Retention Matrix
- Employee Retention-Talent Matrix
- Employee Retention-Turnover Matrix
- Employee Satisfaction Matrix
- Employee Satisfaction-Performance Matrix
- Employee Satisfaction-Value Matrix
- Employee Skill Development Matrix
- Employee Skill Matrix
- Employee Skill-Will Matrix
- Employee Skills-Value Matrix
- Employee Succession Planning Matrix
- Employee Training and Development Matrix
- Employee Training Matrix
- Employee Turnover-Value Matrix
- Engagement-Conversion Matrix
- Engagement-Opportunity Matrix
- Enterprise Architecture Capability Assessment Matrix
- Enterprise Architecture Capability-Value Alignment Matrix
- Enterprise Architecture Capability-Value Proposition Alignment Matrix
- Enterprise Architecture Matrix
- Enterprise Architecture Maturity Matrix
- Enterprise Risk Management Matrix
- Environment-Performance Matrix
- Environment-Social Matrix
- Environmental Analysis Matrix
- Environmental Impact-Performance Matrix
- Environmental Performance-Cost Matrix
- Environmental Risk-Value Matrix
- Environmental Sustainability-Performance Alignment Matrix
- Environmental Sustainability-Value Alignment Matrix
- Ethics and Compliance Maturity Matrix
- Event Planning Matrix
- Experience Mapping Matrix
- Experience Matrix
- Failure Analysis Matrix
- Failure Mode and Effects Analysis (FMEA)
- Failure Mode and Effects Analysis (FMEA) Matrix
- Failure Modes and Effects Analysis (FMEA) Matrix
- Failure Modes and Effects Analysis Matrix
- Feasibility Matrix
- Financial Analysis Matrix
- Financial Performance-Cost Matrix
- Financial Performance-Cost-Value Matrix
- Financial Performance-Performance Alignment Matrix
- Financial Performance-Performance Alignment Matrix
- Financial Performance-Value Matrix
- Financial Performance-Value Proposition Alignment Matrix
- Financial Performance-Value Stream Alignment Matrix
- Financial Planning Matrix
- Financial Statement Analysis Matrix
- FMEA Matrix
- Force Field Analysis Matrix
- Four Corners Analysis
- Franchise Growth-Value Matrix
- Franchise Maturity Matrix
- Franchise Performance-Value Matrix
- Franchise Profitability-Value Matrix
- Franchise Revenue-Value Alignment Matrix
- Franchise Satisfaction-Value Alignment Matrix
- Fraud Risk Matrix
- Friction-Value Matrix
- Frontline Capability Assessment Matrix
- Frontline Capability Development Matrix
- Frontline Performance-Cost Matrix
- Frontline Performance-Cost-Value Matrix
- Future State Operating Model Matrix
- Gap Analysis Matrix
- Gap Analysis-Opportunity Matrix
- Gap Analysis-Performance Matrix
- Gap Analysis-Risk Matrix
- Gartner's Hype Cycle
- GE-McKinsey Matrix
- Geographic Information System Matrix
- Geographic Segmentation Matrix
- Governance Risk-Value Matrix
- Growth Share Matrix
- Growth Strategy Matrix
- Growth Strategy-Value Matrix
- Growth-Share-Size Matrix
- Health and Safety Risk Matrix
- Hoshin Kanri Matrix
- HR Capability Assessment Matrix
- Human Capital Value Matrix
- Idea Evaluation Matrix
- Idea Generation-Evaluation Matrix
- Idea Prioritization Matrix
- Impact Matrix
- Impact-Feasibility Matrix
- Impact-Readiness-Feasibility Matrix
- Incident Management Matrix
- Industry Attractiveness-Competitive Positioning Matrix
- Influencer Marketing Matrix
- Innovation Adoption Matrix
- Innovation and Improvement Matrix
- Innovation and Improvement Matrix
- Innovation and Risk Matrix
- Innovation and Risk Matrix
- Innovation Capability-Performance Alignment Matrix
- Innovation Capability-Value Alignment Matrix
- Innovation Capability-Value Matrix
- Innovation Culture Maturity Matrix
- Innovation Culture-Performance Matrix
- Innovation Ecosystem Matrix
- Innovation Execution Matrix
- Innovation Funnel-Performance Matrix
- Innovation Funnel-Value Alignment Matrix
- Innovation Idea-Value Matrix
- Innovation Management Matrix
- Innovation Maturity Matrix
- Innovation Performance Matrix
- Innovation Performance-Value Alignment Matrix
- Innovation Pipeline Matrix
- Innovation Pipeline-Performance Alignment Matrix
- Innovation Pipeline-Performance Matrix
- Innovation Pipeline-Value Alignment Matrix
- Innovation Pipeline-Value Matrix
- Innovation Pipeline-Value Proposition Alignment Matrix
- Innovation Portfolio Matrix
- Innovation Readiness Matrix
- Innovation Readiness-Value Matrix
- Innovation-Value Proposition Matrix
- Innovation-Value-Complexity Matrix
- Integrated Marketing Communications Matrix
- Intellectual Property Risk Matrix
- Internet of Things Capability-Value Matrix
- Inventory Management Matrix
- Investment Portfolio Matrix
- IT Capability Assessment Matrix
- IT Capability Assessment-Performance Alignment Matrix
- IT Capability Assessment-Value Alignment Matrix
- IT Capability Assessment-Value Matrix
- IT Capability Assessment-Value Proposition Alignment Matrix
- IT Capability Assessment-Value Stream Alignment Matrix
- IT Capability Maturity Matrix
- IT Service Management Capability Assessment Matrix
- IT Service Management Capability Assessment-Value Matrix
- IT Service Management Maturity Matrix
- IT Service Management-Value Matrix
- ITIL Service Capability Matrix
- ITIL Service Value Matrix
- Job Analysis Matrix
- Kano Model Matrix
- Key Performance Indicator (KPI) Matrix
- Key Performance Indicator (KPI) Matrix
- Key Performance Indicators Matrix
- Keyword Research Matrix
- Knowledge Management Capability Matrix
- Knowledge Management Capability-Performance Alignment Matrix
- Knowledge Management Capability-Value Alignment Matrix
- Knowledge Management Capability-Value Proposition-Value Chain Alignment Matrix
- Knowledge Management Matrix
- Knowledge Management Maturity Assessment Matrix
- Knowledge Management Maturity-Performance Matrix
- Knowledge Management Performance-Value Matrix
- Knowledge-Skill Matrix
- Kraljic Matrix
- Ladder of Inference Matrix
- Lead Generation Matrix
- Leadership Capability-Value Matrix
- Leadership Competency Matrix
- Leadership Development Matrix
- Leadership Development-Performance Alignment Matrix
- Leadership Development-Value Alignment Matrix
- Leadership Development-Value Matrix
- Leadership Development-Value Stream Alignment Matrix
- Leadership Performance-Value Alignment Matrix
- Leadership-Value Matrix
- Lean Matrix
- Lean Process Improvement Capability Assessment Matrix
- Lean Process Improvement Capability-Value Alignment Matrix
- Lean Process Improvement Capability-Value Proposition Alignment Matrix
- Lean Process Improvement Matrix
- Lean Process Improvement-Performance Matrix
- Lean Process Matrix
- Lean Process Maturity Matrix
- Lean Six Sigma Capability-Value Matrix
- Life Cycle Cost Analysis Matrix
- Logistics Performance-Value Matrix
- Maintenance Maturity Matrix
- Market Analysis Matrix
- Market Analysis-Performance Alignment Matrix
- Market Analysis-Performance Matrix
- Market Analysis-Size Matrix
- Market Analysis-Value Matrix
- Market Analysis-Value Proposition Alignment Matrix
- Market Attractiveness-Competitive Positioning Matrix
- Market Attractiveness-Competitive Strength Matrix
- Market Attractiveness-Value Matrix
- Market Development Matrix
- Market Development-Growth Matrix
- Market Development-Size Matrix
- Market Environment-Competitive Intensity Matrix
- Market Environment-Market Growth Matrix
- Market Environment-Market Share Matrix
- Market Environment-Market Size Matrix
- Market Environment-Size Matrix
- Market Growth Matrix
- Market Growth-Competitive Positioning Matrix
- Market Opportunity Matrix
- Market Opportunity-Performance Matrix
- Market Opportunity-Size Matrix
- Market Penetration-Performance Matrix
- Market Penetration-Size Matrix
- Market Positioning Matrix
- Market Research Matrix
- Market Segmentation-Performance Alignment Matrix
- Market Segmentation-Performance Matrix
- Market Segmentation-Value Matrix
- Market Segmentation-Value Proposition Alignment Matrix
- Market Share-Growth Matrix
- Market Share-Size Matrix
- Market Size-Growth Matrix
- Market-Value-Complexity Matrix
- Marketing Analytics Matrix
- Marketing Automation Matrix
- Marketing Campaign-Performance Matrix
- Marketing Effectiveness Matrix
- Marketing Effectiveness-Performance Alignment Matrix
- Marketing Effectiveness-Value Alignment Matrix
- Marketing Effectiveness-Value Stream Alignment Matrix
- Marketing Mix Strategy Matrix
- Marketing Mix-Performance Alignment Matrix
- Marketing Mix-Performance Matrix
- Marketing Mix-Value Matrix
- Marketing Performance Matrix
- Marketing Plan Matrix
- Marketing Research Matrix
- Marketing Research-Analysis Matrix
- Materiality Assessment Matrix
- Maturity Assessment Matrix
- Maturity Assessment-Value Matrix
- Maturity Level-Performance Matrix
- Maturity Level-Value Alignment Matrix
- Maturity Level-Value Proposition-Value Chain Alignment Matrix
- Maturity Level-Value Proposition-Value Stream-Value Chain Alignment Matrix
- Maturity Level-Value Proposition-Value Stream-Value Chain-Performance Alignment Matrix
- Maturity Level-Value Stream Alignment Matrix
- Maturity Model Matrix
- Maturity-Value Matrix
- McKinsey 7S Framework
- McKinsey Growth Vector Matrix
- McKinsey Organizational Health Index
- Media Planning Matrix
- Mergers and Acquisitions Matrix
- Messaging-Media Matrix
- Mission-Value-Complexity Matrix
- Mobile Marketing Matrix
- Multi-Criteria Decision Analysis Matrix
- Multichannel Attribution Matrix
- Multichannel Marketing Matrix
- Net Present Value Matrix
- Net Profit Margin Matrix
- Net Promoter Score-Insight Matrix
- Net Promoter Score-Performance Alignment Matrix
- Net Promoter Score-Performance Matrix
- Net Promoter Score-Value Alignment Matrix
- Net Promoter Score-Value Proposition Alignment Matrix
- Net Promoter Score-Value Stream Alignment Matrix
- Net Revenue Matrix
- Net Worth Matrix
- Network Analysis Matrix
- Online Reputation Management Matrix
- Open Innovation Matrix
- Operating Model Capability Assessment Matrix
- Operating Model Capability-Value Matrix
- Operating Model Capability-Value Proposition-Value Chain Alignment Matrix
- Operating Model Matrix
- Operating Model Performance-Cost Matrix
- Operating Model Performance-Value Alignment Matrix
- Operating Model Performance-Value Proposition Alignment Matrix
- Operational Risk Matrix
- Operations Capability-Value Matrix
- Operations Maturity Matrix
- Operations Performance-Value Matrix
- Organic Search-Competitive Positioning Matrix
- Organizational Agility Matrix
- Organizational Agility-Performance Matrix
- Organizational Agility-Value Chain Alignment Matrix
- Organizational Agility-Value Matrix
- Organizational Agility-Value Proposition-Value Stream Alignment Matrix
- Organizational Agility-Value Stream Alignment Matrix
- Organizational Capability Assessment Matrix
- Organizational Capability Assessment-Value Proposition Alignment Matrix
- Organizational Capability Assessment-Value Stream-Value Chain Alignment Matrix
- Organizational Capability Value Matrix
- Organizational Capability-Performance Matrix
- Organizational Capability-Value Alignment Matrix
- Organizational Capability-Value Creation Matrix
- Organizational Capability-Value Proposition Alignment Matrix
- Organizational Capability-Value Proposition-Value Chain Alignment Matrix
- Organizational Capability-Value Stream Alignment Matrix
- Organizational Change Impact Matrix
- Organizational Change Management Maturity Assessment Matrix
- Organizational Change Management Maturity Matrix
- Organizational Change Management-Value Alignment Matrix
- Organizational Change Readiness Matrix
- Organizational Culture Assessment Matrix
- Organizational Culture Maturity Matrix
- Organizational Design-Performance Matrix
- Organizational Design-Value Chain Alignment Matrix
- Organizational Design-Value Matrix
- Organizational Design-Value Proposition-Value Stream Alignment Matrix
- Organizational Design-Value Stream Alignment Matrix
- Organizational Development Maturity Matrix
- Organizational Effectiveness-Value Alignment Matrix
- Organizational Effectiveness-Value Matrix
- Organizational Effectiveness-Value Proposition-Value Chain Alignment Matrix
- Organizational Effectiveness-Value Proposition-Value Stream-Value Chain Alignment Matrix
- Organizational Effectiveness-Value Stream Alignment Matrix
- Organizational Health Assessment Matrix
- Organizational Health Matrix
- Organizational Performance Matrix
- Organizational Performance-Effectiveness Matrix
- Organizational Performance-Value Matrix
- Organizational Readiness-Value Matrix
- Organizational Resilience Matrix
- Organizational Resilience-Performance Matrix
- Organizational Resilience-Value Chain Alignment Matrix
- Organizational Resilience-Value Matrix
- Organizational Resilience-Value Proposition-Value Stream Alignment Matrix
- Organizational Resilience-Value Stream Alignment Matrix
- Organizational Risk-Opportunity Matrix
- Organizational Structure-Value Matrix
- Organizational Sustainability Matrix
- Outsourcing Matrix
- Outsourcing Risk-Value Matrix
- Paid Search-Competitive Positioning Matrix
- Partner Selection Matrix
- Partnership Marketing Matrix
- People Capability Maturity Matrix
- Perception-Positioning Matrix
- Performance and Potential Matrix
- Performance Appraisal Matrix
- Performance Feedback Matrix
- Performance Management Maturity Matrix
- Performance Management-Value Chain Alignment Matrix
- Performance Management-Value Matrix
- Performance Management-Value Proposition-Value Stream Alignment Matrix
- Performance Management-Value Stream Alignment Matrix
- Performance Measurement Matrix
- Performance-Matrix Marketing
- Performance-Value Chain Alignment Matrix
- Performance-Value Matrix
- Performance-Value-Complexity Matrix
- Personality Assessment-Value Matrix
- Personalization Matrix
- PESTLE Analysis Template
- PICK Matrix
- Porter's Five Forces Framework
- Portfolio Analysis Matrix
- Portfolio Analysis-Evaluation Matrix
- Portfolio Analysis-Performance Alignment Matrix
- Portfolio Analysis-Value Alignment Matrix
- Portfolio Assessment-Performance Alignment Matrix
- Portfolio Optimization-Value Alignment Matrix
- Portfolio Optimization-Value Stream Alignment Matrix
- Portfolio Performance-Value Matrix
- Positioning Matrix
- Process Capability Assessment-Value Alignment Matrix
- Process Capability Matrix
- Process Capability-Performance Matrix
- Process Capability-Value Alignment Matrix
- Process Improvement Matrix
- Process Improvement Maturity Matrix
- Process Improvement-Performance Alignment Matrix
- Process Improvement-Value Alignment Matrix
- Process Improvement-Value Stream Alignment Matrix
- Procurement Capability-Value Matrix
- Procurement Maturity Matrix
- Product Adoption Matrix
- Product Costing Matrix
- Product Design Matrix
- Product Design-Development Matrix
- Product Design-Value Matrix
- Product Development Matrix
- Product Development-Reward Matrix
- Product Development-Risk Matrix
- Product Development-Risk-Value Alignment Matrix
- Product Development-Value Matrix
- Product Differentiation Matrix
- Product Feature Matrix
- Product Feature-Value Alignment Matrix
- Product Feature-Value Matrix
- Product Launch-Performance Matrix
- Product Life Cycle Matrix
- Product Portfolio Matrix
- Product Positioning Matrix
- Product Roadmap Matrix
- Product Roadmap-Performance Matrix
- Product-Market Fit Matrix
- Product-Market Matrix
- Product-Market-Technology Matrix
- Product-Service Matrix
- Product-Service Mix Matrix
- Productivity Improvement Matrix
- Program Management Maturity Matrix
- Project Benefit Matrix
- Project Charter Matrix
- Project Management Capability Assessment-Value Matrix
- Project Management Capability-Performance Alignment Matrix
- Project Management Capability-Value Proposition Alignment Matrix
- Project Management Capability-Value Stream Alignment Matrix
- Project Management Matrix
- Project Management Office Maturity Matrix
- Project Management-Performance Alignment Matrix
- Project Prioritization Matrix
- Project Prioritization-Alignment Matrix
- Project Risk Matrix
- Project Risk-Opportunity Matrix
- Project Risk-Value Matrix
- Promotions Matrix
- Prospecting Matrix
- Public Relations Matrix
- Purchase Decision Matrix
- Quadrant Analysis
- Quality Control Matrix
- Quality Control-Performance Matrix
- Quality Function Deployment Matrix
- Quality Management Capability Assessment Matrix
- Quality Management Capability-Value Alignment Matrix
- Quality Management Capability-Value Proposition-Value Chain Alignment Matrix
- Quality Management Matrix
- Quality Management Maturity Matrix
- Quality-Value Matrix
- Rational Decision-Making Matrix
- Recruitment Capability-Value Matrix
- Recruitment Maturity Matrix
- Relationship Management Matrix
- Relationship Marketing Matrix
- Relationship-Value-Complexity Matrix
- Requirements Traceability Matrix
- Resource Allocation Matrix
- Resource Allocation-Utilization Matrix
- Resource Capability Matrix
- Resource Utilization Matrix
- Risk Analysis Matrix
- Risk Assessment Matrix
- Risk Assessment-Value Matrix
- Risk Management Matrix
- Risk Matrix
- Risk Value Matrix
- Risk-Response Matrix
- Risk-Return Matrix
- Roadmap Matrix
- Root Cause Identification Matrix
- Root Cause Matrix
- Sales and Marketing Alignment Matrix
- Sales and Marketing Alignment Matrix
- Sales and Marketing Performance-Value Chain Alignment Matrix
- Sales and Marketing Performance-Value Chain Alignment Matrix
- Sales and Marketing Performance-Value Matrix
- Sales and Marketing Performance-Value Proposition Alignment Matrix
- Sales and Marketing Performance-Value Proposition Alignment Matrix
- Sales and Marketing Performance-Value Proposition-Value Stream Alignment Matrix
- Sales and Marketing-Value Proposition Alignment Matrix
- Sales and Marketing-Value Stream Alignment Matrix
- Sales Capability-Value Matrix
- Sales Effectiveness-Value Matrix
- Sales Force Effectiveness Matrix
- Sales Growth Matrix
- Sales Maturity Matrix
- Sales Performance Matrix
- Sales Performance-Value Alignment Matrix
- Sales Performance-Value Proposition Alignment Matrix
- Sales Performance-Value Proposition-Value Chain Alignment Matrix
- Sales Performance-Value Stream Alignment Matrix
- Sales Performance-Value Stream-Value Proposition Alignment Matrix
- Sales Performance-Volume Matrix
- Sales Pipeline Matrix
- Sales Pipeline-Performance Alignment Matrix
- Sales Pipeline-Value Matrix
- Sales-Value-Complexity Matrix
- Scenario Planning Matrix
- Scrum Agile Matrix
- Search Engine Optimization Matrix
- Security Capability-Value Matrix
- Service Blueprinting Matrix
- Service Capability-Value Matrix
- Service Delivery Capability Assessment-Performance Alignment Matrix
- Service Delivery Capability Assessment-Value Alignment Matrix
- Service Delivery Capability Maturity Assessment-Value Matrix
- Service Delivery Capability Maturity Matrix
- Service Delivery Capability-Value Alignment Matrix
- Service Delivery Matrix
- Service Delivery Performance-Value Alignment Matrix
- Service Delivery Performance-Value Matrix
- Service Delivery-Value Alignment Matrix
- Service Delivery-Value Matrix
- Service Design and Delivery Capability Assessment Matrix
- Service Design and Delivery Matrix
- Service Design and Delivery-Value Alignment Matrix
- Service Design Matrix
- Service Excellence Matrix
- Service Innovation-Performance Alignment Matrix
- Service Innovation-Value Matrix
- Service Innovation-Value Proposition Alignment Matrix
- Service Innovation-Value Stream Alignment Matrix
- Service Management Capability Assessment-Value Matrix
- Service Management Capability Maturity Matrix
- Service Maturity Matrix
- Service Performance-Value Matrix
- Service Profit Chain Matrix
- Service Quality Matrix
- Service-Value-Complexity Matrix
- Six Sigma DMAIC Matrix
- Six Thinking Hats Matrix
- Skill Gap Matrix
- Skill-Will Matrix
- Skills Assessment-Development Matrix
- Skills Assessment-Mapping Matrix
- Skills Assessment-Value Matrix
- Skills Development-Performance Alignment Matrix
- Skills Development-Value Matrix
- Skills Management-Performance Alignment Matrix
- Skills Management-Value Matrix
- Social Capital-Value Matrix
- Social Impact Assessment Matrix
- Social Media Analytics Matrix
- Social Media Capability Maturity Matrix
- Social Media Engagement Matrix
- Social Media Listening-Value Matrix
- Social Media Marketing Matrix
- Social Media Strategy Matrix
- Social Network Analysis Matrix
- Social Responsibility Maturity Matrix
- Social Responsibility-Performance Matrix
- Social Responsibility-Value Chain Alignment Matrix
- Social Responsibility-Value Matrix
- Social Responsibility-Value Proposition Alignment Matrix
- Social Responsibility-Value Stream Alignment Matrix
- Software Development Capability-Value Matrix
- Software Development Lifecycle Matrix
- Software Development Maturity Matrix
- Software Performance-Value Matrix
- Software Quality Matrix
- Software Quality Maturity Matrix
- Software Testing Capability-Value Matrix
- Software Testing Maturity Matrix
- Software-Value-Complexity Matrix
- Solution Design Matrix
- Solution Scalability Matrix
- Sponsorship Marketing Matrix
- Staffing Matrix
- Stakeholder Analysis Grid
- Stakeholder Analysis-Prioritization Matrix
- Stakeholder Analysis-Value Alignment Matrix
- Stakeholder Engagement Matrix
- Stakeholder Management-Value Matrix
- Stakeholder Mapping Matrix
- Stakeholder Mapping-Value Matrix
- Stakeholder Prioritization Matrix
- Stakeholder Prioritization-Value Alignment Matrix
- Stakeholder Risk-Value Alignment Matrix
- Startup Financial Plan Matrix
- Store Atmosphere-Consumer Perception Matrix
- Store Atmosphere-Sales Matrix
- Store Performance Matrix
- Strategic Alignment-Value Matrix
- Strategic Business Unit (SBU) Matrix
- Strategic Business Unit (SBU)-Value Matrix
- Strategic Business Unit (SBU)-Value Matrix
- Strategic Capability-Performance Matrix
- Strategic Capability-Value Chain Alignment Matrix
- Strategic Capability-Value Matrix
- Strategic Capability-Value Proposition Alignment Matrix
- Strategic Capability-Value Proposition-Value Chain Alignment Matrix
- Strategic Capability-Value Stream Alignment Matrix
- Strategic Capability-Value Stream-Value Proposition Alignment Matrix
- Strategic Maturity Matrix
- Strategic Option Matrix
- Strategic Performance-Value Alignment Matrix
- Strategic Performance-Value Chain Alignment Matrix
- Strategic Performance-Value Matrix
- Strategic Performance-Value Proposition-Value Chain Alignment Matrix
- Strategic Performance-Value Stream-Value Chain Alignment Matrix
- Strategy Culture Matrix
- Strategy Execution Capability Matrix
- Strategy Execution-Performance Matrix
- Strategy Map
- Strategy Map Matrix
- Supplier Capability Maturity Matrix
- Supplier Capability-Performance Matrix
- Supplier Performance Matrix
- Supplier Performance-Cost Matrix
- Supplier Performance-Opportunity Matrix
- Supplier Performance-Value Alignment Matrix
- Supplier Relationship Management Matrix
- Supplier Risk-Value Matrix
- Supplier Sustainability Performance-Cost Matrix
- Supplier Sustainability Performance-Value Alignment Matrix
- Supply Chain Analysis Matrix
- Supply Chain Capability-Value Matrix
- Supply Chain Complexity Matrix
- Supply Chain Efficiency Matrix
- Supply Chain Integration-Value Alignment Matrix
- Supply Chain Optimization-Value Alignment Matrix
- Supply Chain Performance-Value Matrix
- Supply Chain Risk Matrix
- Supply Chain Risk-Performance Matrix
- Supply Chain Risk-Value Matrix
- Supply Chain Visibility Matrix
- Supply Chain Visibility-Cost Matrix
- Sustainability Performance-Value Chain Alignment Matrix
- Sustainability Performance-Value Matrix
- Sustainability Performance-Value Proposition Alignment Matrix
- SWOT Analysis Matrix for Marketing
- SWOT-Gap Matrix
- SWOT-PEST Analysis Matrix
- System Development Capability-Value Matrix
- System Development Maturity Matrix
- System Performance-Value Matrix
- Systems Engineering Capability-Value Matrix
- Systems Engineering Maturity Matrix
- Talent Acquisition-Performance Matrix
- Talent Assessment-Value Alignment Matrix
- Talent Development Matrix
- Talent Development-Performance Matrix
- Talent Management Capability-Value Alignment Matrix
- Talent Management Matrix
- Talent Maturity Matrix
- Talent Performance-Value Alignment Matrix
- Talent Performance-Value Matrix
- Talent Pool Matrix
- Talent Review Matrix
- Talent-Value-Complexity Matrix
- Target Market Matrix
- Technology Adoption Lifecycle
- Technology Assessment Matrix
- Technology Capability Assessment Matrix
- Technology Capability Matrix
- Technology Capability Maturity Assessment-Value Matrix
- Technology Capability Maturity Model
- Technology Capability-Value Alignment Matrix
- Technology Capability-Value Proposition Alignment Matrix
- Technology Evaluation Matrix
- Technology Innovation Matrix
- Technology Innovation-Value Matrix
- Technology Innovation-Value Proposition Alignment Matrix
- Technology Innovation-Value Stream Alignment Matrix
- Technology Maturity Matrix
- Technology Maturity-Value Matrix
- Technology Performance-Value Alignment Matrix
- Technology Performance-Value Matrix
- Technology Platform Matrix
- Technology Risk-Opportunity Matrix
- Technology Risk-Performance Matrix
- Technology Risk-Value Alignment Matrix
- Technology Roadmap Matrix
- Technology Strategy Matrix
- Technology Strategy-Value Matrix
- Technology Strategy-Value Proposition Alignment Matrix
- Technology Strategy-Value Proposition-Value Chain Alignment Matrix
- Technology-Value-Complexity Matrix
- Test Matrix
- The 7 Habits of Highly Effective People
- The Impact-Effort Matrix
- Time Management Matrix
- Time Value of Money Matrix
- Time-Performance-Value Matrix
- Total Cost of Ownership-Value Alignment Matrix
- Total Cost of Quality-Value Alignment Matrix
- Total Quality Management Matrix
- Total Quality Management Maturity Matrix
- Total Value Chain Alignment Matrix
- Total Value Matrix
- TOWS Analysis Matrix
- Trade Show Marketing Matrix
- Training Capability-Value Matrix
- Training Effectiveness-Performance Alignment Matrix
- Training Evaluation Matrix
- Training Impact Matrix
- Training Maturity Matrix
- Training Needs Assessment Matrix
- Training Needs Assessment-Value Alignment Matrix
- Training Needs-Performance Alignment Matrix
- Training Performance-Value Matrix
- Training-Value-Complexity Matrix
- Transition Capability-Value Matrix
- Transition Maturity Matrix
- User Experience Matrix
- User Experience-Value Matrix
- User Experience-Value Proposition Matrix
- User Experience-Value Proposition-Value Chain Alignment Matrix
- User Experience-Value Stream Alignment Matrix
- User Needs-Value Proposition Alignment Matrix
- User Research-Value Proposition Alignment Matrix
- Value Chain Analysis
- Value Chain Analysis Matrix
- Value Chain-Performance Matrix
- Value Creation Matrix
- Value Mapping Matrix
- Value Proposition Canvas
- Value Proposition-Competitive Advantage Matrix
- Value Stream Mapping Capability Maturity Matrix
- Value Stream Mapping Matrix
- Value-Based Pricing Matrix
- Vendor Evaluation Matrix
- Vendor Management-Performance Matrix
- Vendor Performance-Cost Matrix
- Vendor Performance-Value Alignment Matrix
- Vendor Risk Matrix
- Vendor Risk-Cost Matrix
- Vendor Risk-Performance Alignment Matrix
- Viral Marketing Matrix
- Voice of Customer Matrix
- Website Traffic-Conversion Matrix
- Work Breakdown Structure (WBS)
- Workforce Capability Development-Performance Alignment Matrix
- Workforce Capability Development-Value Alignment Matrix
- Workforce Capability-Performance Matrix
- Workforce Capability-Value Alignment Matrix
- Workforce Development-Performance Alignment Matrix
- Workforce Development-Value Matrix
- Workforce Development-Value Proposition Alignment Matrix
- Workforce Performance-Value Chain Alignment Matrix
- Workforce Performance-Value Proposition-Value Chain Alignment Matrix
- Workforce Performance-Value Stream Alignment Matrix
- Workforce Skill Development Matrix
- YouTube Marketing Matrix
What is the Data Science Maturity Matrix?
A visual explanation is shown in the image above. The Data Science Maturity Matrix can be described as a matrix with the following quadrants:
- Ad Hoc Analysis: Isolated data science projects with limited organizational impact. Example: A single department conducting ad hoc analysis.
- Operational Analytics: Data science integrated into business operations for decision-making. Example: Real-time operational dashboards used across departments.
- Basic Reporting: Basic data collection and reporting with minimal analytics. Example: Regularly scheduled reports generated from collected data.
- Advanced Predictive Analytics: Enterprise-wide adoption of advanced analytics for strategic insights. Example: Predictive models driving business strategy across the organization.
What is the purpose of the Data Science Maturity Matrix?
The Data Science Maturity Matrix is a framework designed to help organizations evaluate their data science capabilities and maturity. It is typically represented as a 2x2 matrix, with the x-axis representing the level of data sophistication (from basic data collection to advanced analytics) and the y-axis representing the level of organizational integration (from isolated projects to enterprise-wide adoption).
Use cases for the Data Science Maturity Matrix include:
- Assessment: Organizations can use the matrix to assess their current data science capabilities and identify areas for improvement.
- Strategic Planning: The matrix provides a roadmap for organizations to advance their data science maturity, helping them prioritize investments and initiatives.
- Benchmarking: Companies can benchmark their data science maturity against industry standards or competitors.
- Communication: The matrix serves as a communication tool to align stakeholders on the current state and future direction of data science initiatives.
By understanding their position on the matrix, organizations can develop targeted strategies to enhance their data science capabilities, drive innovation, and achieve better business outcomes.
Want to try this template?
What templates are related to Data Science Maturity Matrix?
The following templates can also be categorized as business, data science, analytics and are therefore related to Data Science Maturity Matrix: Product-Market Matrix, 4 Ps Marketing Mix Matrix, AI Capability-Value Proposition Alignment Matrix, AI Innovation-Value Alignment Matrix, AI Maturity Matrix, AI-Value Proposition Alignment Matrix, AI-Value Proposition Matrix, AIDA Marketing Matrix. You can browse them using the menu above.
How can I use Data Science Maturity Matrix in Priority Matrix?
You can get Data Science Maturity Matrix in your Priority Matrix in just a moment:
- Click to sign in or create an account in the system
- Start adding your items to the matrix
- If you prefer it, download Priority Matrix and take your data with you
Learn more about Data Science Maturity Matrix, and get free access to lots of other templates, at templates.app. Once you are comfortable with the document, you can easily export to Excel, if you prefer to work that way.
If you have any questions and you can't find the answer in our knowledge base, don't hesitate to contact us for help.